Model-Prime Accelerates Four Growers’ Greenhouse Robotics
A company at the forefront of automated greenhouse crop management, Four Growers was founded in 2018 with a mission to revolutionize farming and agriculture. Their autonomous robots navigate greenhouse rows and swiftly select ripe produce for harvest at the right time, reducing labor costs and enabling the supply of healthy, affordable local produce to consumers.
Four Growers robots collect and analyze vast amounts of valuable data daily. Leveraging this data, robot performance is continually improved, and greenhouses can learn about their production enabling them to increase their yields.
The challenge: Overcoming bottlenecks in robot data management
Four Growers robots’ capacity to collect valuable data on crops demands the right technology to process it and engineers to work with it. Analyzing robot logs, retrieving specific information, and performing other crucial data-related tasks are often time-consuming, manual processes. Four Growers sought a software solution to address data management bottlenecks in their workflow.
Struggles with log search and retrieval
The Four Growers engineering team stores robot data logs in the cloud, but native cloud storage search didn’t allow them to easily find the data they needed to support their troubleshooting and development processes. Engineers weren’t able to filter data based on crucial attributes like location, weather conditions, or software version, and instead had to search for them manually, looking through folders and comparing timestamps — a tedious process, especially when dealing with an increasing fleet size. Hence, finding robot logs was time-consuming and cumbersome and slowed down tasks like data analysis or troubleshooting.
Limited data analysis and context
After retrieving the logs they needed, Four Growers lacked a system to enrich them with additional insights. While logs can capture valuable sensor data, they need human context for interpretation. Observations during testing or production runs weren’t captured alongside logs, making it difficult to interpret the data quickly and accurately. As a workaround, engineers managed this information in spreadsheets, which resulted in significant manual effort and time investment. As such, transforming raw data into actionable insights was a time-consuming process and left engineers with little room to track trends and identify performance issues.
Lastly, analyzing the downloaded log files added another layer of complexity. Engineers were forced to download the files and load them individually into a dedicated simulation environment to review information about the logs or to analyze results, consuming valuable engineering time and adding another step to an already inefficient workflow.
Inability to find interesting events
Like many robotics companies, Four Growers faced challenges in managing and extracting insights from their extensive log data. They lacked an efficient way to identify critical portions of their logs, which significantly hindered their ability to make informed decisions quickly.
Four Growers was particularly focused on understanding when and why errors occurred in the field. This understanding was crucial for prioritizing both developer and operations resources effectively and delivering an exceptional customer experience.
Previously, their process involved using spreadsheets to track time periods of interest, searching for the log encompassing that time, and then downloading entire logs to inspect or running scripts on the data—a step they referred to as “data preparation.” However, this approach was time-consuming and could not scale with the organization’s growth and increasing data generation. Solving this challenge became essential for maintaining operational efficiency and supporting continued growth.
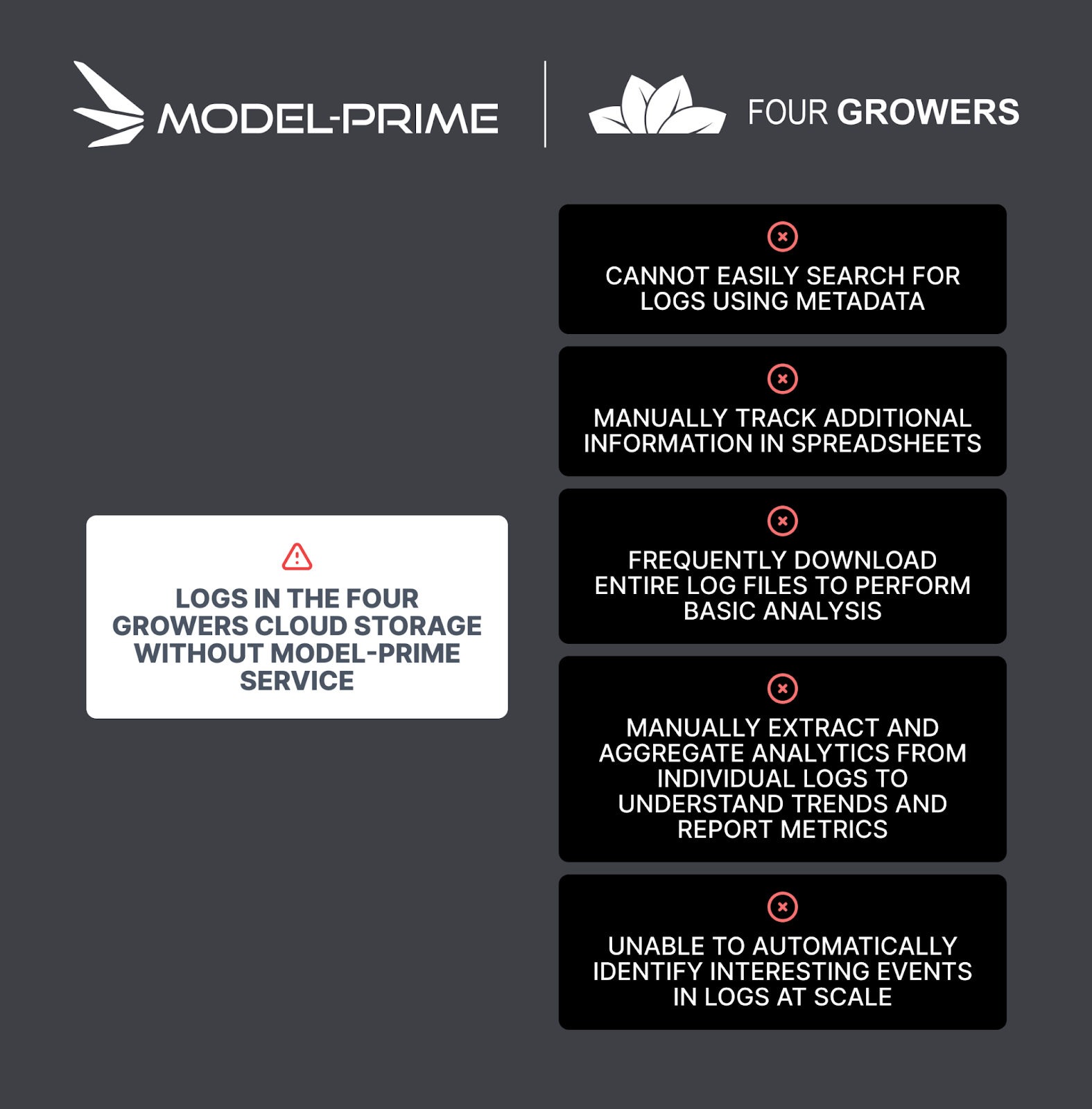
The Solution: Model-Prime’s platform saves time and adds depth to log analysis
Model-Prime provided Four Growers with a cloud-based data platform specifically designed to address robotics data challenges. Adopting Model-Prime’s secure and scalable cloud platform allowed Four Growers to unlock a new level of data management and solve the challenges it was facing thanks to a multi-pronged solution.
Robot logs annotation and search plugin
To reduce manual efforts to understand and locate relevant log files, Model-Prime provided an intuitive web-based application that enabled Four Growers engineers to browse through and search a catalog of its log files. Engineers can quickly view results from robot runs, search for logs based on patterns of interest, and further enrich their log data with additional insights.
In this case, Model-Prime provided a user-friendly interface so engineers can view their entire log catalog, allowing them to:
- Effortlessly find the right logs: Quickly filter and search based on key attributes like robot type, region, season, and software version instead of tedious folder navigation.
- Implement native annotations: View and add crucial contextual notes made by operators during testing or production runs. No more missing insights due to absent human observations.
- Avoid downloading log files: The ability to browse and analyze log data without the need to download files and individually load them into a simulation environment.
This intuitive system streamlined log search and retrieval, saving Four Growers a staggering 5-10x in time compared to their previous methods. Now, valuable insights are just a click away, critical for faster decision-making.
Model-Prime also provides API access so Four Growers can integrate internal applications to help capture performance annotations in the field and write them to the log.
Automated Event Tagging
Identifying critical events in log data at scale is essential for organizations like Four Growers to leverage their data and make informed decisions effectively. By implementing Model-Prime’s event auto-tagging feature, Four Growers could automatically isolate log segments where errors occurred, significantly reducing the time spent on data preparation and manual log inspection.
This capability allowed Four Growers to quickly diagnose the root causes of errors in the field and quickly identify the conditions under which these errors arose. Additionally, the auto-tagging functionality enabled them to build dynamic dashboards for error tracking, significantly improving precision and visibility.
As a result, Four Growers eliminated their reliance on spreadsheets for error tracking, streamlining their workflows and allowing their team to focus on optimizing field operations. This shift empowered them to make faster, data-driven decisions, enhancing operational efficiency and driving software progress forward.
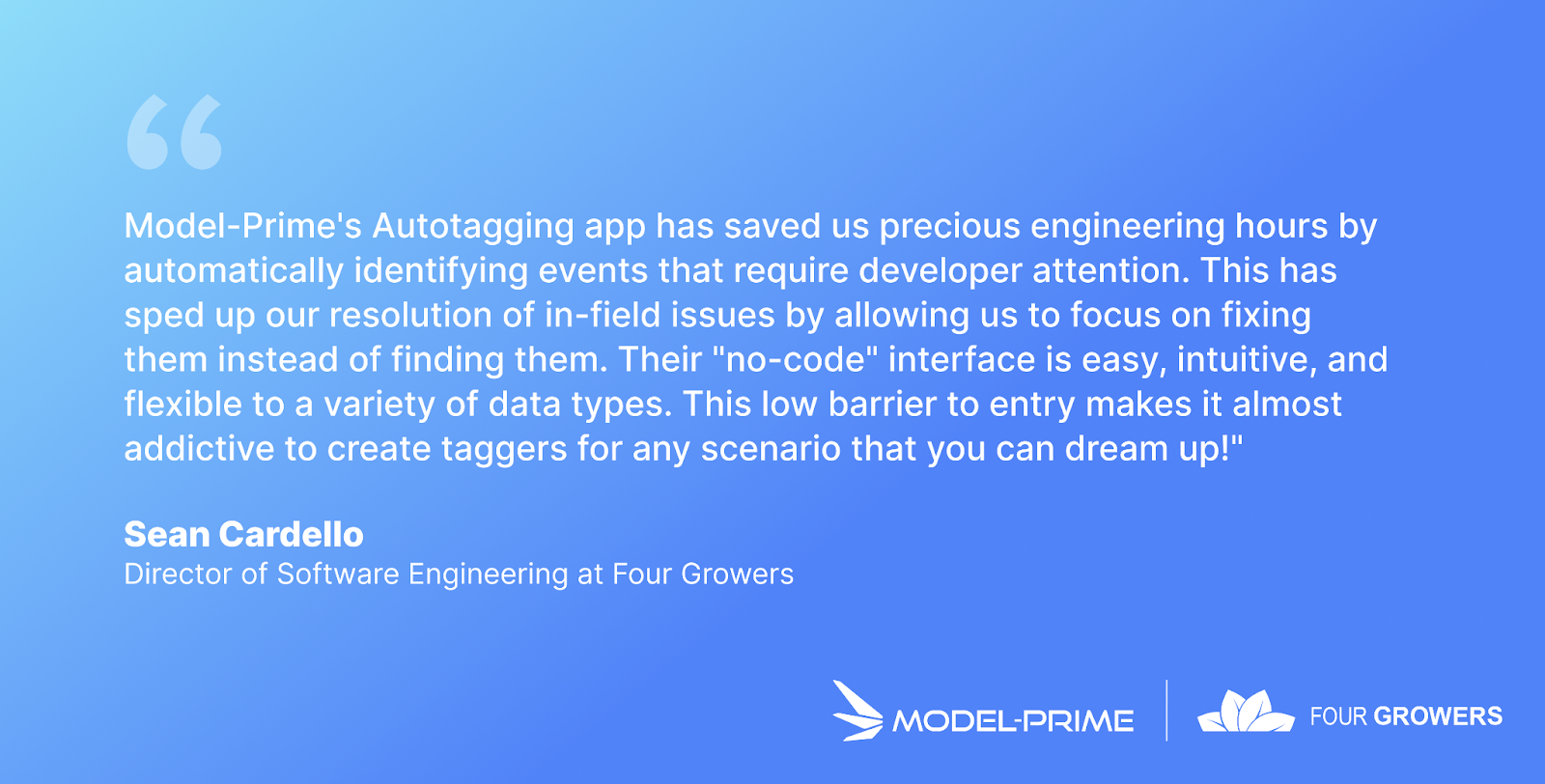
Impact Summary
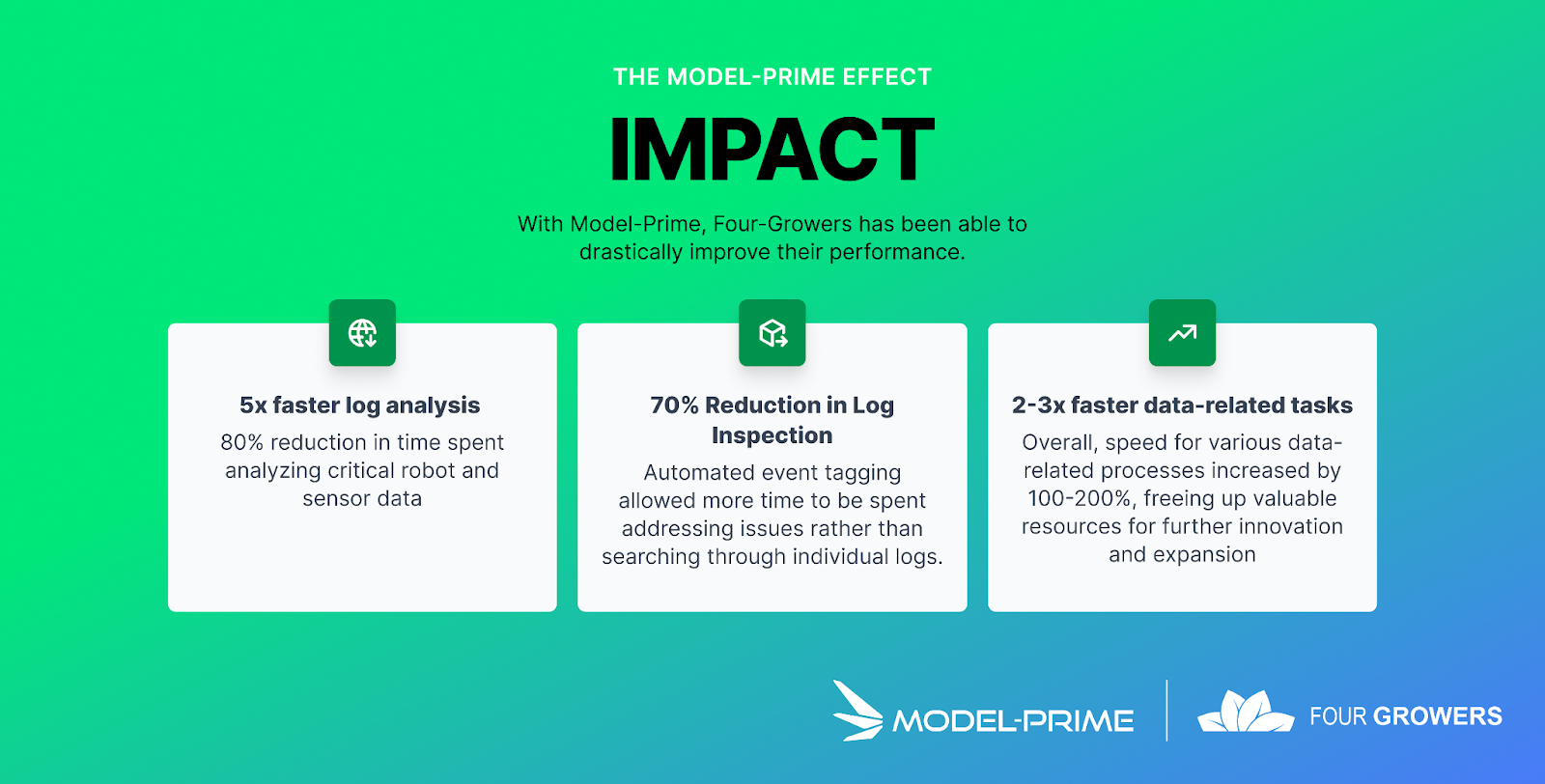